NEXT STORY
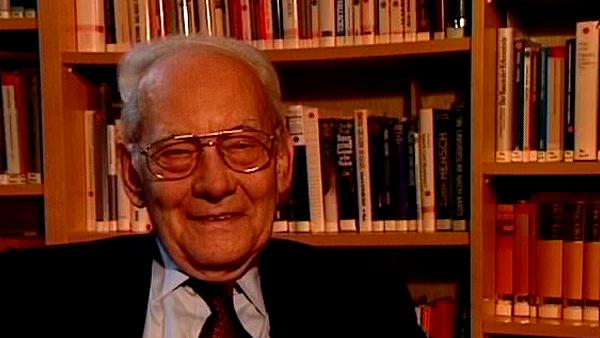
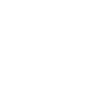
The space of mutants
RELATED STORIES
NEXT STORY
The space of mutants
RELATED STORIES
![]() |
Views | Duration | |
---|---|---|---|
61. Extending Darwinian theory to molecules | 157 | 01:31 | |
62. The difference between my theory and classical genetic theory | 227 | 02:31 | |
63. The fitness landscape | 231 | 02:18 | |
64. The complexity of a sequence of nucleotides | 122 | 03:37 | |
65. Population and mutation | 118 | 01:22 | |
66. The progress of evolution | 122 | 02:47 | |
67. Finding a ridge in sequence space | 108 | 01:25 | |
68. Low and high dimensional landscapes | 116 | 02:47 | |
69. Peter Schuster's concept of sequence space and shape space | 133 | 04:34 | |
70. The space of mutants | 109 | 01:27 |
He treated it with an example, an example which you can treat quantitatively. He looked at nucleic acids, RNA, ribonucleic acids, and asked for the pairing of the molecules on the base pairing rules. You know, we have talked already about these building blocks of nucleic acids that they make positive and negative strands; in other words, the purine likes a given pyrimidine to pair with, the A with the U or T, the G with the C. And so he said, 'Given a random sequence, what is the chance... how much mutations do I have to introduce to make a completely paired molecule out of it?' That means that parts of the molecule must be complementary to one another. And he said that, 'I rate that as the highest fitness value'. That's something he could calculate.
And... now if you think of a molecule of lengths of 100... well, if you would have only two classes of building blocks then you would on average have to change 50 ones; in other words 50 ones you can take as given and for them you have to find the complementary ones. If you do that with nucleic acids you would have to change 75, you could only say that one quarter is the correct, the others you have to test. So you see that looks a very pretty difficult job, to find the best, in this case the best paired sequence. He found that what you have to do is to change on average 10 bases and you get there. So he developed the concept not only of a sequence space but also for a shape space. And you could go on and could say a shape space, if you look at proteins, the shape will determine a function of the protein molecule so you can have [define it as] a function space. And in order to get there you don't have to test all possibilities. Think of a molecule, a very small molecule of 100 bases. If you would have to test through them... through all possibilities, you have 4100 different sequences. 4100. That's 1060. So that's more molecules than you can accommodate on earth... on the surface on earth. So, if you would rely entirely on chance in testing those molecules, you would be lost, you wouldn't get there. But he found out that is not the case, you can do so and you can show that this is a way. He did computer experiments with it, and you see the progress, you get a mutant, and suddenly it stays for a long time and then it goes on again.
Now 'it stays for long time' does not mean nothing is happening in the population. We talked about neutral mutants, we talked about those at the periphery which are almost, or as good as, the in the centre of gravity. Well, this population fluctuates now. It might settle around one which formerly was on the periphery. You don't see that in the fitness landscape because it's neutral. So when it goes on again it's distribution has changed so much that the entirely new distribution came about in which progress again is possible. So in other words many things happen while you are on the same level due to neutral migration.
By the way, this is one aspect... also the population genetics theory has come about. Kimura has developed a theory of neutral mutations where he says that the main progress in evolution has been due to neutral mutants.
Nobel Prize winning German biophysical chemist, Manfred Eigen (1927-2019), was best known for his work on fast chemical reactions and his development of ways to accurately measure these reactions down to the nearest billionth of a second. He published over 100 papers with topics ranging from hydrogen bridges of nucleic acids to the storage of information in the central nervous system.
Title: Peter Schuster's concept of sequence space and shape space
Listeners: Ruthild Winkler-Oswatitch
Ruthild Winkler-Oswatitsch is the eldest daughter of the Austrian physicist Klaus Osatitsch, an internationally renowned expert in gas dynamics, and his wife Hedwig Oswatitsch-Klabinus. She was born in the German university town of Göttingen where her father worked at the Kaiser Wilhelm Institute of Aerodynamics under Ludwig Prandtl. After World War II she was educated in Stockholm, Sweden, where her father was then a research scientist and lecturer at the Royal Institute of Technology.
In 1961 Ruthild Winkler-Oswatitsch enrolled in Chemistry at the Technical University of Vienna where she received her PhD in 1969 with a dissertation on "Fast complex reactions of alkali ions with biological membrane carriers". The experimental work for her thesis was carried out at the Max Planck Institute for Physical Chemistry in Göttingen under Manfred Eigen.
From 1971 to the present Ruthild Winkler-Oswatitsch has been working as a research scientist at the Max Planck Institute in Göttingen in the Department of Chemical Kinetics which is headed by Manfred Eigen. Her interest was first focused on an application of relaxation techniques to the study of fast biological reactions. Thereafter, she engaged in theoretical studies on molecular evolution and developed game models for representing the underlying chemical proceses. Together with Manfred Eigen she wrote the widely noted book, "Laws of the Game" (Alfred A. Knopf Inc. 1981 and Princeton University Press, 1993). Her more recent studies were concerned with comparative sequence analysis of nucleic acids in order to find out the age of the genetic code and the time course of the early evolution of life. For the last decade she has been successfully establishing industrial applications in the field of evolutionary biotechnology.
Tags: Neutral theory of molecular evolution, purine, pyrimidine, ribonucleic acid, RNA, sequence space, shape space, fitness landscape, neutral mutants, neutral migration, population genetics, Peter K Schuster, Motoo Kimura
Duration: 4 minutes, 35 seconds
Date story recorded: July 1997
Date story went live: 24 January 2008